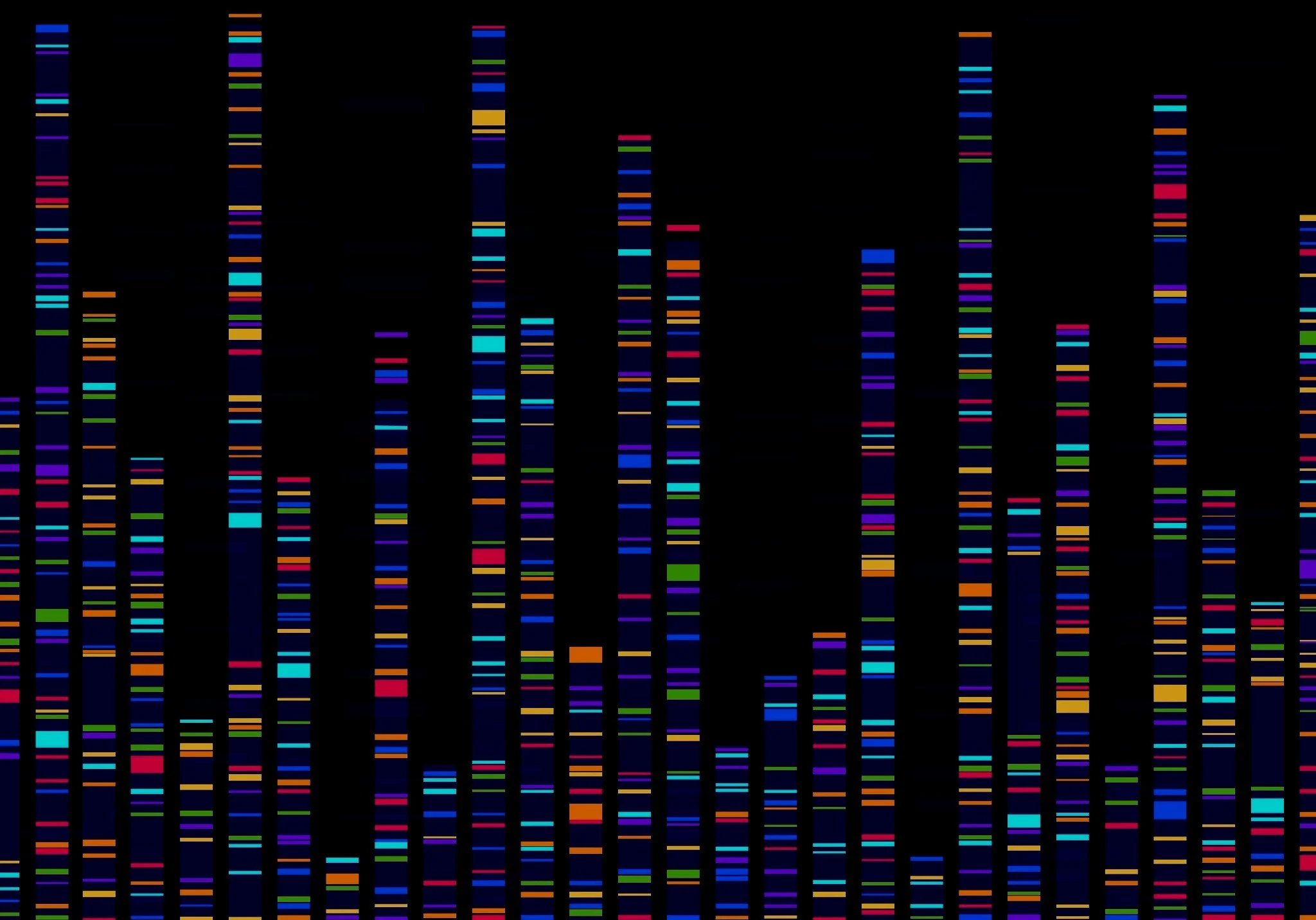
Biostatistics Problem Set for Pre-clinical Gene Therapy Trials
Here is a sample problem set we solved for one of our clients working on testing a pre-clinical gene therapy drug
Background: You are designing a preclinical study to test several interventions in a disease model.
There are 7 animal groups: 'healthy control', 'disease vehicle control', and 5 'disease + therapeutic intervention' groups. The effect size for the primary disease outcome between 'healthy control' and 'disease control' is 2.3. We aim to see a 50% recovery of this effect size for disease control vs. disease intervention groups. The study power is 80%. All variables are continuous variables. Several types of measurements will be performed during the study including:
Type 1 measurement: Made at multiple time points including baseline, midpoints, and post-treatment.
Type 2 measurement: Measurements made at baseline and post-treatment.
Type 3 measurement: Measurements made only at the endpoint.
Questions:
What statistical test would you use to determine if any interventions show efficacy for measurement types 1, 2, and 3?
Please specify which groups you would include/exclude for determining statistical significance and your rationale for the choice of test. Based on this study design, how would you perform a power analysis?
Our solution (An excerpt of the work)
What statistical test would you use to determine (if) any of the interventions shows efficacy for measurement types 1, 2, and 3?
Please specify which groups you would include/exclude for determining statistical significance and your rationale for the choice of test.
Type 1 Measurements: Multiple Time Points (Baseline, Midpoints, Post-Treatment)
METHOD 1
Statistical test: Repeated Measures ANOVA
Assumption: We can quantify a particular phenotype the drug affects and accurately measure it over time. This is the dependent variable.
Additional test-linked assumptions:
The dependent variable should be continuous and normally distributed
The independent variable (number of categorical ‘matched pairs’) should be at least two. For instance, we have three or more - baseline, midpoint, and post-treatment.
The variances of the differences between all possible pairs of repeated measures should be equal (for the within-subjects factor i.e. across different time points).
Groups to be included: The five 'disease + therapeutic intervention' groups
Rationale: Repeated measures ANOVA will allow you to assess within-subject effects over time. This test will help us understand if any treatments worked over time. A posthoc test such as Tukey HSD can be performed to understand which therapeutics worked (For instance, it will tell you which of the five therapeutic interventions showed improvements over time)
METHOD 2
Statistical test: Mixed Design ANOVA
Assumption: We can quantify a particular phenotype the drug affects and accurately measure it over time. This is the dependent variable.
Additional test-linked assumptions:
The dependent variable should be continuous and normally distributed.
The independent variable (number of categorical ‘matched pairs’) should be at least two. For instance, we have three or more - baseline, midpoint, and post-treatment.
The variances of the differences between all possible pairs of repeated measures should be equal (for the within-subjects factor i.e. across different time points).
The variances of the different groups should be equal (for the between-subjects factor).
Groups to be included: All 7 groups (healthy control, disease vehicle control, and five disease + therapeutic intervention groups).
Rationale: Mixed Design ANOVA will allow you to assess within-subject effects over time as well as between-subject effects. It examines the effect of time or condition on the dependent variable within the same subjects (within-subjects factor) and compares differences between groups (between-subjects factor). A posthoc test such as Tukey HSD can be performed to understand which therapeutics worked (For instance, it will tell you which of the five therapeutic interventions showed improvements over time compared to the diseased or healthy control)
Type 2 Measurements: Two Time Points (Baseline and Post-Treatment)
Statistical test: Two-way repeated measures ANOVA (on two-time points, the rest of the points are identical to the ones mentioned above)
Assumption: We can quantify a particular phenotype the drug affects and accurately measure it over time. This is the dependent variable.
Additional test-linked assumptions:
The dependent variable should be continuous and normally distributed
The independent variable (number of categorical ‘matched pairs’) should be at least two. For instance, we have three or more - baseline, midpoint, and post-treatment.
The variances of the differences between all possible pairs of repeated measures should be equal (for the within-subjects factor i.e. across different time points).
Groups to be included: The five 'disease + therapeutic intervention' groups
Rationale: Repeated measures ANOVA will allow you to assess within-subject effects over time. This test will help us understand if any treatments worked over time. A posthoc test such as Tukey HSD can be performed to understand which therapeutics worked (For instance, it will tell you which of the five therapeutic interventions showed improvements over time)
Other tests that will also work:
Paired t-test
One-way ANOVA: Can use the change scores (Post-treatment measurement - Baseline measurement) and assign those to the appropriate group i.e. healthy, disease vehicle control, or one of the disease + therapeutic intervention groups. Then perform a regular one-way ANOVA using all seven groups.
Type 3 Measurements: Single Time Point (Measurements made only at the endpoint)
Statistical test: One-way ANOVA
Assumption: We can quantify a particular phenotype the drug affects and accurately measure it over time. This is the dependent variable.
Additional test-linked assumptions:
The dependent variable should be continuous and normally distributed
The independent variable (number of categorical ‘matched pairs’) should be at least two. For instance, we have three or more - baseline, midpoint, and post-treatment.
The variances of the differences between all possible pairs of repeated measures should be equal (for the within-subjects factor i.e. across different time points).
Groups to be included: All 7 groups (healthy control, disease vehicle control, and five disease + therapeutic intervention groups)
Rationale: One-way ANOVA will determine if there are any significant differences in the endpoint measurements among the groups. Post-hoc tests (e.g., Tukey's HSD) would follow if the ANOVA shows significant results.
NOTE: In case any of the stated assumptions are not met by the data, we can always use a non-parametric test such as Kruskal-Wallis instead of ANOVA.
Based on this study design, how would you perform a power analysis?
So we wish to calculate the sample size to detect a given effect size with a specified level of power and significance level. To do so, we need the following -
The effect size f
The level of significance, α
Power (1 - β)
Number of groups (G)
But, we have been given the effect size we aim to detect → 50% of 2.3 = 0.05*2.3 = 1.15
We have three types of experiments, Type 1, Type 2, and Type 3, each potentially requiring a different approach to calculate the effect size.
Using an online calculator, I found f = 0.468 (using aimed effect size and number of groups).
Next, we can plug all these values per test -
Type 1 - One-way ANOVA:
Effect Size (f): 0.468
Number of Groups: 7
Type 2 - Two-way ANOVA (Group x Time):
Effect Size (f): 0.468
Number of Groups: 7
Levels of Time: 2 (baseline and post-treatment)
Type 3 - Repeated Measures ANOVA:
Effect Size (f): 0.468
Number of Groups: 7
Number of Measurements: depends on the time points (e.g., 3 for baseline, midpoints, post-treatment)
We can use a software such as G*Power to input these parameters and calculate the required sample sizes for each analysis type.